DOE EERE: METS-R
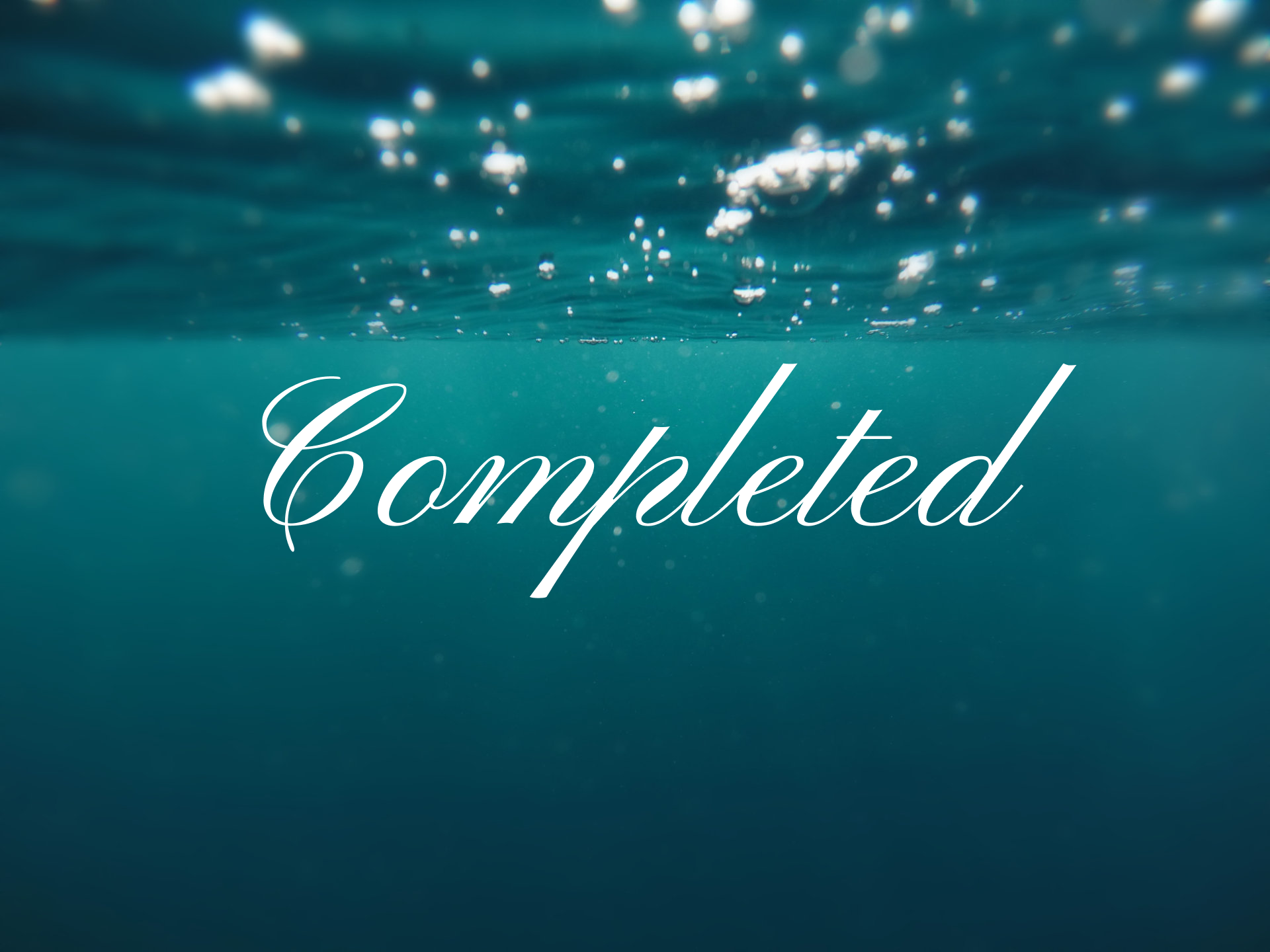
- Principal Investigator: Satish Ukkusuri, Purdue University
- Co-Principal Investigator: Stanislav Sobolevsky, New York University
- Co-Principal Investigator: Milind Kulkarni, Purdue University
- Sponsor: DOE - U.S. Department of Energy
- Duration: 10/2018-10/2020
There have been unprecedented changes in urban transportation in the last few years. A wide range of mobility services (e-hailing, ridesharing) offers new kinds of transportation alternatives that connect various users in a mobility as a service (MaaS) eco-system. Despite the rapid technology advances and the availability of large-scale information systems, many urban commuters still rely on transportation systems designed decades ago to fulfill their daily travel needs. Several inefficiencies in the transportation system exist, including low usage rate of public transportation modes and excessive private vehicle ownership. This leads to wasted vehicle capacity, heavier congestion and emissions, and, therefore, unnecessary energy consumption. In particular, transportation hubs such as railway terminals and airports suffer most from these inefficiencies, since they bring together a large number of commuters in short time spans from multiple modes and are sub-optimal at leveraging multi-modal mobility services. Passenger trips at urban transportation hubs have several distinct characteristics that differ from urban commuting trips, and specialized solution approaches are required in order to develop an energy-optimal travel platform for efficiently serving these passengers. First of all, trips to-and-from a transportation hub share the same trip origin or destination: the hub itself. This provides an opportunity to promote ridesharing at transportation hubs so that the total number of trips, and therefore energy consumption, may be significantly reduced. Second, multiple transportation modes are available at transportation hubs, and it is therefore important to optimally balance the usage of existing modes to achieve optimal energy use. Third, the arrival of passengers at transportation hubs depends highly on the timetable (of trains and flights) at the hubs, thereby leading to more predictable demand, which makes it more convenient to optimize trip scheduling in real-time. Finally, compared to regular commuting trips, some passengers at transportation hubs are less mobile due to the luggage they carry and special needs and have special characteristics in terms of their travel mode choice. The conjunction of these issues presents unique challenges that should be addressed. Currently, while some analysis has been done on mode specific mobility needs there are no studies which quantify the energy impacts of these multi-modal mobility platforms. The goal of this project is to develop the next generation travel solution at urban transportation hubs to substantially reduce transportation energy usage. Passengers have diverse travel needs at transportation hubs, so that energy consumption from a multi-modal perspective is especially important in our proposal. We will combine data acquisition techniques with energy saving automated electric vehicles(AEV) to design a data-driven smart transportation mode as a supplement to existing travel modes to optimize energy flow at transportation hubs. The team will leverage high-performance computing (HPC) clusters to develop an advanced simulation-based platform to support and validate real-time energy optimal trip scheduling, to achieve impactful travel time and energy savings.
Publications
- Lei et al. (2024). METS-R SIM: A simulator for Multi-modal Energy-optimal Trip Scheduling in Real-time with shared autonomous electric vehicles. Simulation Modelling Practice and Theory, 132, 102898.
- Qian et al. (2020). Impact of transportation network companies on urban congestion: evidence from large-scale trajectory data. Published in Sustainable Cities and Society.
- Stanislav Sobolevsky et. al. (2019). Anomaly detection in temporal networks. NetSci, May, 2019, Burlington, VT, USA.
- Qian et al. (2019). Stationary spatial charging demand distribution for commercial electric vehicles in urban area. IEEE ITSC 2019.
- Lei et al. (2019). Optimal proactive vehicle relocation for on-demand mobility service with deep 3convolution-lstm network. IEEE ITSC 2019.
- Qian et al. (2019). Demand-adaptive transit design for urban transportation hubs. Accepted for presentation at 2020 TRB annual meeting.
- Qian et al. (2019). Charging Infrastructure Planning For Commercial Electric Vehicles Based on Stationary Spatial Demand Distribution. Accepted for presentation at 2020 TRB annual meeting.
- Qian et al. (2019). Understand the impact of transportation network companies on urban traffic using large-scale trajectory data. Accepted for presentation at 2020 TRB annual meeting.